- AI Goodies ⨠The Newsletter
- Posts
- šš AI for UX Research
šš AI for UX Research
How to employ AI in our research process. The tools, opportunities, limitations and dangers.
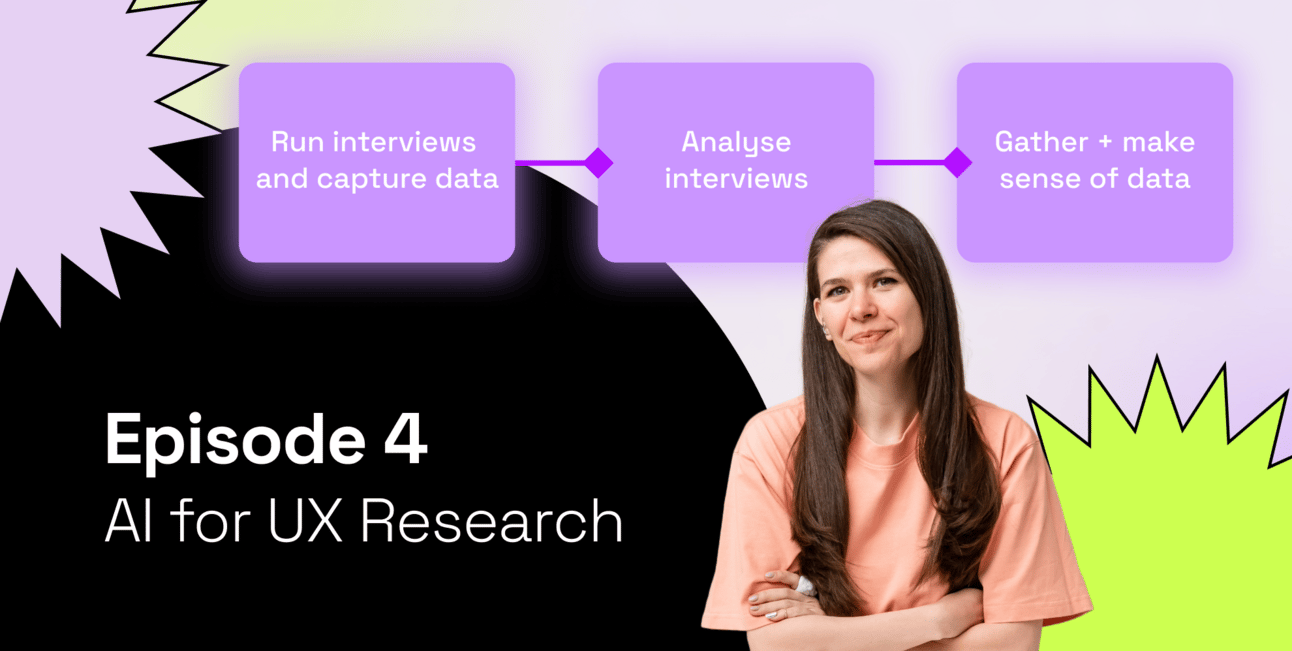
Episode 4: AI for UX research šš
Welcome back to the AI Goodies community! š
Research feels like that last area that AI would be well-positioned to replace, but it many ways it is the one it can support best. AI technologies are good for a couple of things, and making sense of large volumes of data is one of them.
But when you think about involving AI in research a couple of questions immediately pop up:
āWhat are the tools and scenarios that I can confidently employ?ā
āCan I trust AI to produce and interpret research data?ā
āIs it ethical?ā
Weāll be looking into what are the opportunities AI presents us with when it comes to research and how to navigate this tricky problem space in an ethical, safe way.
ā ļø Caution: The Limitations of these tools
We know that AI is still infant technology, so we are mostly aware that it can easily produce inaccurate results. But thereās much more to worry us when it comes to research. A couple of problems:
Lack of citations & accuracy of information: When generating quotes and recommendations, some tools fail to reference their sources, being unable to attribute quotes or link them to specific clips. In a recent study by Nielsen Norman the tools they tested couldnāt distinguish between the researcherās notes and the session transcript, which raises important ethical issues since it's crucial to differentiate between interpretations and participant statements.
Furthermore, this lack of citation prevents researchers from verifying if the toolās summaries or recommendations are based on actual research.
AI is biased: The biases of AI has can be divided in these categories: systematic, statistical, computational, and human. Read more about Open AI efforts to reduce the biases of ChatGPT.
AI lacks context: Most AI-powered research tools donāt ask for, and canāt accept things such as the study goals, insights and tags from previous research, background information about the users, and so on. This lack of a broader context can lead to a multitude of research failures. For example, with no understanding of the research goals, the tool canāt know what is important or relevant to the researcher.
Current AI tools offer vague insights and recommendations: The actions proposed by these tools usually are vague and lack clarity, so theyāre mostly not actionable.
On top of all these points, thereās also the problem of user research tools over-promising, creating false expectations while delivering often unreliable results.
One of the best article to dive into these limitations is this research by the NN Group.
š„ Some great AI tools for your research process
The most popular slide from my AI talk! It essentially imagines and E2E design process augmented by AI at stages where that makes sense. Of course, the key lens through which to look at it is that you are still at the center of orchestrating all of this.
If we look at the first line, we can interpret it in the context of discovery and research work, and I want to invite you all to an exercise: think about the activities that go into your research efforts (maybe better to write them down) and then start exploring if and how they might get supported (safely) with the help of AI.

Do you use AI tools in your UX research?Out of all the tools mentioned above, or other ones - do you use any tools in your day to day UX process? |
Below I share a couple of use-cases that Iām exploring in detail in my course about AI for Designers.
š Use case #1: AI for Market Research & Competitive Analysis
Yes, youāve read it right! We can use ChatGPT or Gemini to gather preliminary information on consumer needs, trends & key behaviours. (ā ļø Just make sure to fact check all information that it provides).
How do we do that?
Ask the following questions from ChatGPT to get to know your productās target market better, letās find out the most common problems they face.
Your product: Productivity mobile app
āI would like to understand the most common problems that people face when it comes to everyday productivity. What are their common struggles?ā

The results that ChatGPT generated
Of the results generated above, you could narrow down the topic for more relevant results:
āI would like to learn more about procrastination. Can you expand on this?ā
We can also use ChatGPT to help us carry out a Competitive analysis.
Letās ask:
āAre there any apps currently helping people with the problem of procrastination?ā
āWhat is the most popular productivity app right now?ā
šāāļø Use case #2: AI-powered User Interviews
You can essentially use AI in two ways to support you with user interviews:
Generate an interview script based on your research goals and questions
Create AI-generated personas (risky and with important ethical considerations to bear in mind)
Tools to use: ChatGPT, Gemini, Synthetic Users
ChatGPT can support you with a good draft for your user interview guide. If you want to explore ābraverā applications of AI around interviews, you can try out a (controversial but interesting!) tool called Synthetic Users.
I personally think it can be an useful tool that can complement nicely real user interviews. Imagine you have a set of insights out of which some have not been sufficiently validated or explored, and are working under time and resource constrains. You can tap into the power of these AI-constructed archetypes, essentially manifestations of your representative user, and have an interesting dialogue with an entity constructed based on the demographic and statistical information you feed it.
ā ļø Caution! These personas may not fully reflect reality. They also donāt cover users with disabilities, with specific edge cases needs, as AI provides the average user persona. Make sure to validate the information provided with real people. Never leave out real user testing.
šŖļø Use case #3: Data processing & synthesis
AI can be very useful in helping you process large sets of data and notice trends & key information. It can also extract learnings from your interview notes & recordings and provide suggested actions.
Tableau: Offers data visualisation and business intelligence tools with built-in AI features for data analysis.
Microsoft Power BI: Has the ability to sort through data and visualise it to search for insights.
H2O.ai: Provides an open-source AI platform for machine learning and AI-driven data analysis.
Miro AI: Miro Assist can support you in research synthesis: bring all the data on your board and then use AI to help you process and distill it into the essential points and transform insights into shareable formats.
ChatGPT: (or) you can simply paste interview transcripts into ChatGPT and ask it to extract the key insights.
š§ Use case #4: Usability testing with AI-powered Tools
There are various tools that you can use to predict user performance based on past data, generate predictive heat maps, provide UX audit or help synthesise large sets of data.
Attention Insight: This tool predicts where users will look after engaging with your design. Apart from predictive heat maps, it can help you compare designsāyou can use alternate versions of the same design or even a competitorās design to evaluate how well your interface performs.
Predict by Neurons: generates predictive heatmaps of where your audience might look at your designs and has very similar features to Attention Insight. The main difference between the two tools lies in the AIās underlying ātrainingā. While Attention Insight bases its predictions on eye-tracking studies, Predict uses data from eye-tracking and neuroscientific experiments.
3Mās Visual Attention System (VAS): This tool is similar to the above tools and extends to physical environments in addition to digital interfaces.
Heurix: A heuristic analysis tool that conducts a UX audit of a website and automatically generates a report highlighting the areas of improvement.
UserTesting AI: Helps synthesise large volumes of video, text and behavioural data to identify key themes, patterns and insights. The toolās sentiment analysis feature focuses on what users say during a testing session. Its friction detection focuses on behavioural cues (what users actually do) to pinpoint the exact places where participants had difficulty interacting with websites or prototypes during tests.
š Final thoughts
These tools can offer a great helping hand for researchers. However, the AI-augmented research process shouldnāt miss the humanity of actually talking to users, seeing their emotions while testing products & discussing how it makes them truly feel.
š„ Letās collaborate: Would you like to appear in my newsletter?
Check out my sponsor deck to find out details about how your brand can reach itās potential with my Newsletterās audience šš¼
⨠Relevant articles
š Next on AI Goodies: Episode 5 - Brainstorming with AI
In the next newsletter I will cover the ways in which AI can help us Brainstorm product ideas & solutions for product problems.
Send this Newsletter to a friend who would be interested, link below šš¼
⨠For more Goodies, follow me on:
AI & Design Love, Ioana ā„ļø